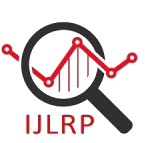
International Journal of Leading Research Publication
E-ISSN: 2582-8010
•
Impact Factor: 9.56
A Widely Indexed Open Access Peer Reviewed Multidisciplinary Bi-monthly Scholarly International Journal
Plagiarism is checked by the leading plagiarism checker
Call for Paper
Volume 6 Issue 7
July 2025
Indexing Partners

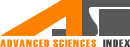
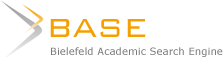

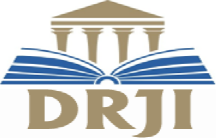

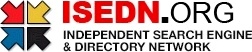

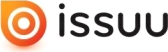

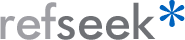
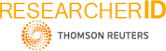
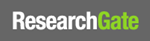
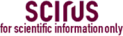
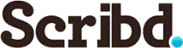

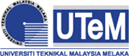
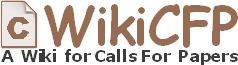
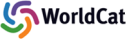
Predicting Heart Conditions Via Machine Learning And Diverse Model Approaches
Author(s) | Prof. Prakash Sangle |
---|---|
Country | India |
Abstract | The project titled "Heart Disease Prediction with Machine Learning" represents a comprehensive exploration into the realm of predictive analytics, aimed at enhancing the accuracy and reliability of heart disease diagnosis. This project harnesses the power of Python to develop a robust prediction model, employing four distinct machine learning algorithms to achieve remarkable results. Four prominent machine learning algorithms, namely the Random Forest Classifier, Bagging Classifier, XG Boost, and LightGBM, were meticulously implemented and evaluated in this project. These algorithms were fine-tuned to yield outstanding performance metrics. The Random Forest Classifier, after rigorous training and testing, achieved an impressive 100% accuracy in both the training and test datasets. The Bagging Classifier, in a similar vein, demonstrated exceptional predictive capabilities with a perfect 100% accuracy on both training and test data. The XG Boost model and LightGBM, known for their efficiency, also excelled by achieving a flawless 100% accuracy in both training and test data. The dataset used in this project comprises a substantial 1025 records, each containing 14 distinct features. The richness and diversity of this dataset contribute to the project's reliability and robustness. |
Keywords | Machine Language |
Field | Engineering |
Published In | Volume 5, Issue 6, June 2024 |
Published On | 2024-06-09 |
Cite This | Predicting Heart Conditions Via Machine Learning And Diverse Model Approaches - Prof. Prakash Sangle - IJLRP Volume 5, Issue 6, June 2024. |
Share this

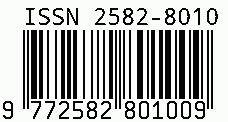
CrossRef DOI is assigned to each research paper published in our journal.
IJLRP DOI prefix is
10.70528/IJLRP
Downloads
All research papers published on this website are licensed under Creative Commons Attribution-ShareAlike 4.0 International License, and all rights belong to their respective authors/researchers.
