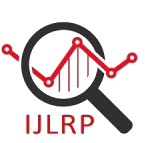
International Journal of Leading Research Publication
E-ISSN: 2582-8010
•
Impact Factor: 9.56
A Widely Indexed Open Access Peer Reviewed Multidisciplinary Bi-monthly Scholarly International Journal
Plagiarism is checked by the leading plagiarism checker
Call for Paper
Volume 6 Issue 4
April 2025
Indexing Partners

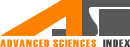
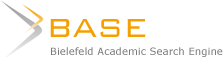

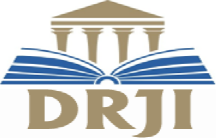

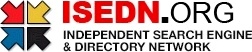

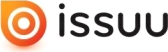

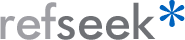
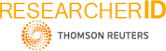
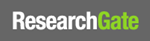
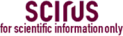
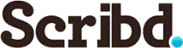

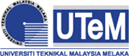
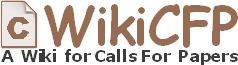
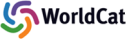
Design of a Deep Learning-Based Hybrid Image Caption Generation Method
Author(s) | T Sravanthi, Mr B Javeed Basha |
---|---|
Country | India |
Abstract | In this observe, we behavior an in-intensity analysis of a deep neural network-based totally picture captioning method. Given an input photo, the method can generate an English sentence that describes the content of the picture. We observe the 3 major components of this approach: sentence technology, recurrent neural networks (RNNs), and convolutional neural networks (CNNs). We find that with the aid of changing the three modern structures with the CNN factor, the VGG Net network performs better in terms of BLEU score. As a brand new recurrent layer, we additionally advise a simplified version of gated recurrent gadgets (GRU) that may be carried out in Caffe using both MATLAB and C++. Compared to the long short-term memory (LSTM) technique, the simplified GRU produces similar results. However, it has fewer parameters, which reduces reminiscence usage and speeds up studying. Finally, we use beam search to generate multiple propositions. Experiments show that the up to date approach uses much less memory for schooling and produces up-to-date signatures with modern technology. |
Keywords | Recurrent Neural Networks (RNN), Convolutional Neural Networks (CNN), Gated Recurrent Gadgets (GRU), Long Short-Term Memory (LSTM) |
Field | Engineering |
Published In | Volume 6, Issue 4, April 2025 |
Published On | 2025-04-14 |
Cite This | Design of a Deep Learning-Based Hybrid Image Caption Generation Method - T Sravanthi, Mr B Javeed Basha - IJLRP Volume 6, Issue 4, April 2025. |
Share this

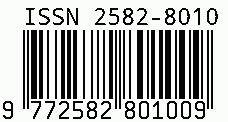
CrossRef DOI is assigned to each research paper published in our journal.
IJLRP DOI prefix is
10.70528/IJLRP
Downloads
All research papers published on this website are licensed under Creative Commons Attribution-ShareAlike 4.0 International License, and all rights belong to their respective authors/researchers.
