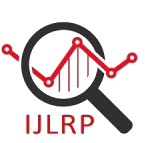
International Journal of Leading Research Publication
E-ISSN: 2582-8010
•
Impact Factor: 9.56
A Widely Indexed Open Access Peer Reviewed Multidisciplinary Bi-monthly Scholarly International Journal
Plagiarism is checked by the leading plagiarism checker
Call for Paper
Volume 6 Issue 4
April 2025
Indexing Partners

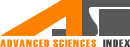
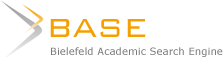

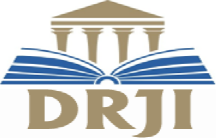

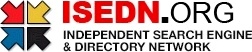

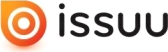

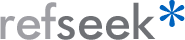
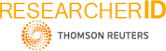
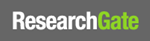
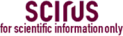
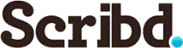

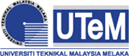
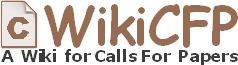
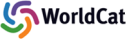
AI-Powered Drug Discovery Accelerating Pharmaceutical Research and Development
Author(s) | Praveen Kumar Rawat |
---|---|
Country | United States |
Abstract | AI-driven techniques for drug development have transformed pharmaceutical R&D by significantly shortening the time and minimizing expenditures in evaluating prospective drug candidates. While classical drug discovery is highly experimental-dependent, AI-based procedures use machine learning (ML) and ensemble algorithms to optimize the predicting of molecular properties, drug-target interactions, and virtual screening. This paper discusses the ensemble learning techniques, including Bagging, Boosting (XGBoost, LightGBM), and Stacking, to provide greater accuracy and reliability in drug discovery. Bagging reduces variance by averaging many predictions received from several models, whereas Boosting focuses on areas poorly predicted by previous weak learners and iteratively improves learning by minimizing residual errors. Stacking offers gains in prediction accuracy by combining multiple base models, each trained independently, using a meta-learner. These ensemble-based approaches gain particular significance in drug-likeness predictions, toxicity considerations, and optimization of clinical trials. Other deep learning ensembles, as opposed to boosting algorithms or stacking ensembles modeled, the Convolutional Neural Network (CNN) or Graph Neighborhood Graph Neural Network (GNN) can accurately determine several molecular interactions while significantly spontaneous lead optimization and drug-repurposing strategies. Such AI models combined with ML allow generative design of drugs with optimal molecular structures in view of pharmacokinetic properties, depending on Reinforcement Learning (RL). AI-powered drug discovery contributes to efficient hypothesis generation, clinical trial failure rates diminution, and an accelerated pace of innovation in pharmaceuticals by leveraging large-scale biochemical datasets. The present study emphasizes the power of ensemble machine learning in drug discovery and suggests an AI-based framework for future pharmaceutical innovative developments. |
Keywords | Ensemble Learning, Machine Learning, Drug-Target Interactions, Virtual Screening, Boosting, Deep Learning And Pharmaceutical Research |
Field | Medical / Pharmacy |
Published In | Volume 6, Issue 3, March 2025 |
Published On | 2025-03-11 |
Cite This | AI-Powered Drug Discovery Accelerating Pharmaceutical Research and Development - Praveen Kumar Rawat - IJLRP Volume 6, Issue 3, March 2025. DOI 10.5281/zenodo.15125089 |
DOI | https://doi.org/10.5281/zenodo.15125089 |
Short DOI | https://doi.org/g9bnk9 |
Share this

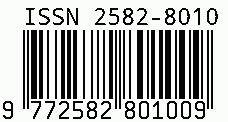
CrossRef DOI is assigned to each research paper published in our journal.
IJLRP DOI prefix is
10.70528/IJLRP
Downloads
All research papers published on this website are licensed under Creative Commons Attribution-ShareAlike 4.0 International License, and all rights belong to their respective authors/researchers.
