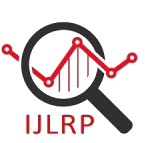
International Journal of Leading Research Publication
E-ISSN: 2582-8010
•
Impact Factor: 9.56
A Widely Indexed Open Access Peer Reviewed Multidisciplinary Bi-monthly Scholarly International Journal
Plagiarism is checked by the leading plagiarism checker
Call for Paper
Volume 6 Issue 4
April 2025
Indexing Partners

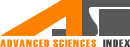
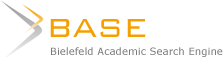

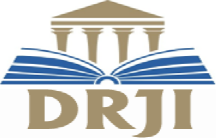

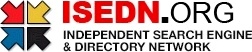

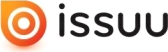

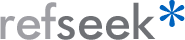
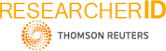
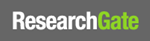
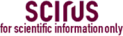
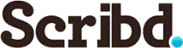

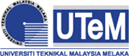
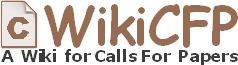
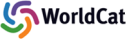
Automating Data Quality Assurance Using Machine Learning in ETL Pipelines
Author(s) | Raghavender Maddali |
---|---|
Country | India |
Abstract | The Rising data processing pipeline complexity and size necessitate the use of secure automated means to ensure data quality during Extract, Transform, Load (ETL) processes. This article presents a machine learning framework that is capable of automating data quality assurance in ETL pipelines using anomaly detection, predictive modeling, and self-healing. The framework leverages machine learning algorithms to detect inconsistencies, predict potential data errors, and autonomously correct issues, enhancing data integrity, accuracy, and consistency in real-time processing. By continuously monitoring data quality, the system reduces manual intervention, minimizes operational costs, and improves decision-making reliability. The framework is adaptable across various industries, including healthcare, finance, and manufacturing, where high-quality data is essential for business intelligence and analytics. Experimental findings reveal that it successfully minimizes data errors and strengthens the ETL pipeline significantly. The suggested approach offers a scalable and smart solution to ensure high-quality data in a changing environment of data. |
Keywords | ETL automation, data quality assurance, machine learning, anomaly detection, predictive modeling, self-healing mechanisms, real-time data processing, data integrity. |
Field | Computer > Data / Information |
Published In | Volume 2, Issue 6, June 2021 |
Published On | 2021-06-08 |
Cite This | Automating Data Quality Assurance Using Machine Learning in ETL Pipelines - Raghavender Maddali - IJLRP Volume 2, Issue 6, June 2021. DOI 10.5281/zenodo.15107533 |
DOI | https://doi.org/10.5281/zenodo.15107533 |
Short DOI | https://doi.org/g8986j |
Share this

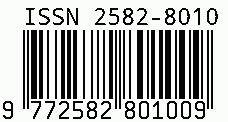
CrossRef DOI is assigned to each research paper published in our journal.
IJLRP DOI prefix is
10.70528/IJLRP
Downloads
All research papers published on this website are licensed under Creative Commons Attribution-ShareAlike 4.0 International License, and all rights belong to their respective authors/researchers.
