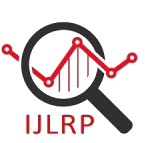
International Journal of Leading Research Publication
E-ISSN: 2582-8010
•
Impact Factor: 9.56
A Widely Indexed Open Access Peer Reviewed Multidisciplinary Bi-monthly Scholarly International Journal
Plagiarism is checked by the leading plagiarism checker
Call for Paper
Volume 6 Issue 4
April 2025
Indexing Partners

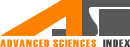
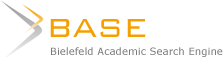

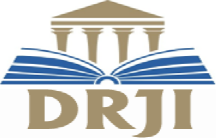

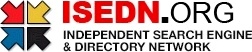

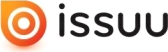

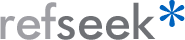
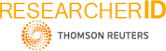
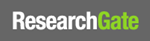
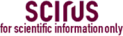
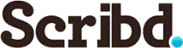

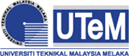
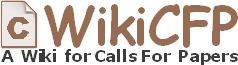
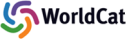
Reinforcement Learning-Based Data Pipeline Optimization for Cloud Workloads
Author(s) | Raghavender Maddali |
---|---|
Country | India |
Abstract | The Use of machine learning (ML) and reinforcement learning (RL) techniques towards optimizing cloud workload, resource allocation, and data processing efficiency. Recent developments in ML-based models such as hybrid workload predictive models, data storage technology using deep learning techniques, and secure offloading architectures have brought excellent developments in cloud and fog computing environments. Reinforcement learning has also worked exceptionally well in network scheduling optimization, query engine optimization, and parallelism for big data. ML-based solutions have been used to provide solutions for low-latency problems in memory management, load balance, and multistep multi-workflow scheduling in data centers. This paper emphasizes the application of ML and RL for optimizing cloud workload to help achieve adaptive scalability, cost, and better usage of resources and some of them as intelligent systems to build workload forecasts, pipeline optimization for data, and fair resource allocation to have more scalable and effective cloud computing configuration. |
Keywords | Cloud Workload Prediction, Reinforcement Learning, Resource Provisioning, Big Data Processing, Machine Learning, Workload Optimization, Data Center Networks, Parallelization, Load Balancing, Query Engine Optimization, Fog-Cloud Computing, Adaptive Scalability, Low Latency, Cost Efficiency. |
Field | Computer > Data / Information |
Published In | Volume 1, Issue 1, September 2020 |
Published On | 2020-09-03 |
Cite This | Reinforcement Learning-Based Data Pipeline Optimization for Cloud Workloads - Raghavender Maddali - IJLRP Volume 1, Issue 1, September 2020. DOI 10.5281/zenodo.15107752 |
DOI | https://doi.org/10.5281/zenodo.15107752 |
Short DOI | https://doi.org/g8988d |
Share this

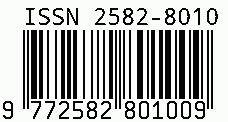
CrossRef DOI is assigned to each research paper published in our journal.
IJLRP DOI prefix is
10.70528/IJLRP
Downloads
All research papers published on this website are licensed under Creative Commons Attribution-ShareAlike 4.0 International License, and all rights belong to their respective authors/researchers.
