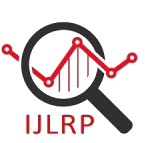
International Journal of Leading Research Publication
E-ISSN: 2582-8010
•
Impact Factor: 9.56
A Widely Indexed Open Access Peer Reviewed Multidisciplinary Bi-monthly Scholarly International Journal
Plagiarism is checked by the leading plagiarism checker
Call for Paper
Volume 6 Issue 4
April 2025
Indexing Partners

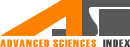
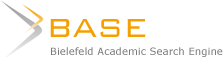

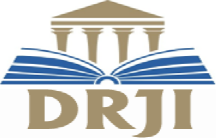

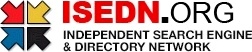

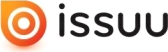

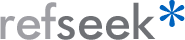
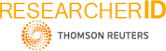
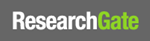
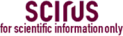
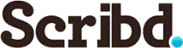

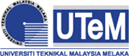
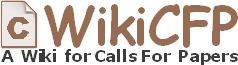
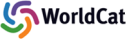
Unsupervised Learning for High-Dimensional Data: Advancements in Unsupervised Learning Techniques like Clustering, Anomaly Detection, and Dimensionality Reduction
Author(s) | Gaurav Kashyap |
---|---|
Country | United States |
Abstract | In the field of machine learning, unsupervised learning has become an essential tool for analyzing high-dimensional, complex data. Unlike supervised learning, which relies on labeled data for model training, unsupervised learning methods aim to identify hidden patterns and structures in unlabeled data. Recent developments in unsupervised learning methods are examined in this paper, with an emphasis on dimensionality reduction, anomaly detection, and clustering. These methods are essential for effective data analysis because high-dimensional data, sometimes known as the "curse of dimensionality," poses serious difficulties for conventional machine learning algorithms. We go over the development of these techniques, their uses, difficulties, and the most recent advancements in the field of high-dimensional data handling. In the age of high-dimensional data, unsupervised learning techniques have grown in importance. This study explores the developments in dimensionality reduction, anomaly detection, and clustering techniques for intricate, high-dimensional datasets. It examines the unique challenges posed by such data and the innovative approaches that have emerged to tackle them. |
Keywords | Unsupervised Learning, High-Dimensional Data, Clustering, Anomaly Detection, Dimensionality Reduction, Curse of Dimensionality, Pattern Recognition, Data Analysis, Feature, Extraction, Autoencoders, Principal Component Analysis (PCA), t-Distributed Stochastic Neighbor, Embedding (t-SNE), Manifold Learning, Deep Learning, Data Visualization, Subspace Clustering, Outlier, Detection, Nonlinear Dimensionality Reduction, Spectral Clustering, Local Outlier Factor (LOF), Graph Convolutional Networks (GCN) |
Field | Engineering |
Published In | Volume 5, Issue 12, December 2024 |
Published On | 2024-12-04 |
Cite This | Unsupervised Learning for High-Dimensional Data: Advancements in Unsupervised Learning Techniques like Clustering, Anomaly Detection, and Dimensionality Reduction - Gaurav Kashyap - IJLRP Volume 5, Issue 12, December 2024. DOI 10.5281/zenodo.14866681 |
DOI | https://doi.org/10.5281/zenodo.14866681 |
Short DOI | https://doi.org/g84xqg |
Share this

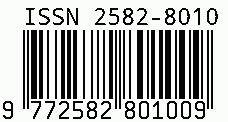
CrossRef DOI is assigned to each research paper published in our journal.
IJLRP DOI prefix is
10.70528/IJLRP
Downloads
All research papers published on this website are licensed under Creative Commons Attribution-ShareAlike 4.0 International License, and all rights belong to their respective authors/researchers.
