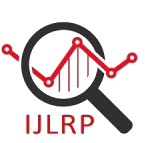
International Journal of Leading Research Publication
E-ISSN: 2582-8010
•
Impact Factor: 9.56
A Widely Indexed Open Access Peer Reviewed Multidisciplinary Bi-monthly Scholarly International Journal
Plagiarism is checked by the leading plagiarism checker
Call for Paper
Volume 6 Issue 4
April 2025
Indexing Partners

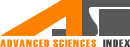
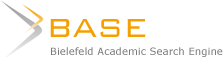

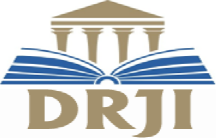

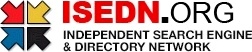

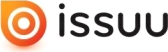

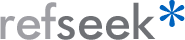
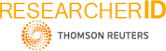
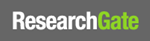
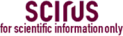
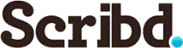

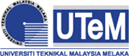
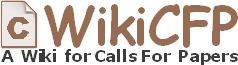
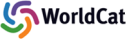
Leveraging Unsupervised Learning for Identifying Unknown Defects in New Semiconductor Products
Author(s) | Tarun Parmar |
---|---|
Country | United States |
Abstract | Semiconductor manufacturing faces significant challenges in terms of identifying and resolving unknown defects during the introduction of new products. Traditional root cause analysis methods struggle to detect and classify novel defect types, leading to delays, increased cost, and potential quality issues. Unsupervised learning has emerged as a promising approach for addressing these challenges by automatically discovering patterns, anomalies, and relationships within large, complex datasets without the need for predefined labels. This review explores the potential of unsupervised learning techniques, such as clustering algorithms, dimensionality reduction, and anomaly detection, to enhance the root cause analysis in semiconductor manufacturing. Case studies demonstrated the effectiveness of methods such as principal component analysis, k-means clustering, autoencoders, and generative adversarial networks in grouping similar defect patterns, identifying rare defects, visualizing high-dimensional data, and uncovering hidden correlations. However, challenges remain in handling high-dimensional data, addressing imbalanced datasets, interpreting results, and integrating the domain knowledge. Future research directions include combining unsupervised and supervised learning, incorporating temporal data, applying deep learning to defect image analysis, and developing real-time monitoring systems. Integrating unsupervised learning with domain expertise promises to significantly improve the quality control efficiency and effectiveness in semiconductor manufacturing, ultimately leading to faster time-to-market, reduced costs, and improved product quality for new semiconductor devices. |
Keywords | semiconductor manufacturing, root cause analysis, unknown defects, unsupervised learning, clustering algorithms, anomaly detection |
Field | Engineering |
Published In | Volume 1, Issue 3, November 2020 |
Published On | 2020-11-10 |
Cite This | Leveraging Unsupervised Learning for Identifying Unknown Defects in New Semiconductor Products - Tarun Parmar - IJLRP Volume 1, Issue 3, November 2020. DOI 10.5281/zenodo.14840180 |
DOI | https://doi.org/10.5281/zenodo.14840180 |
Short DOI | https://doi.org/g84hh6 |
Share this

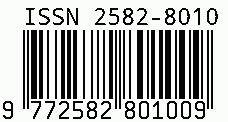
CrossRef DOI is assigned to each research paper published in our journal.
IJLRP DOI prefix is
10.70528/IJLRP
Downloads
All research papers published on this website are licensed under Creative Commons Attribution-ShareAlike 4.0 International License, and all rights belong to their respective authors/researchers.
