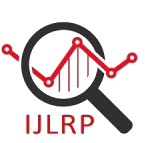
International Journal of Leading Research Publication
E-ISSN: 2582-8010
•
Impact Factor: 9.56
A Widely Indexed Open Access Peer Reviewed Multidisciplinary Bi-monthly Scholarly International Journal
Plagiarism is checked by the leading plagiarism checker
Call for Paper
Volume 6 Issue 4
April 2025
Indexing Partners

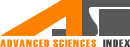
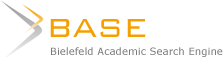

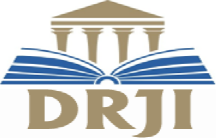

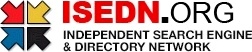

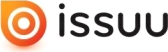

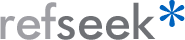
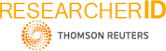
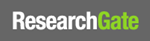
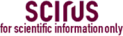
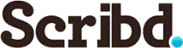

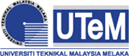
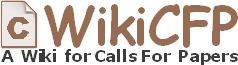
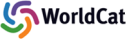
An Overview of Model Compression Techniques for Deep Neural Networks
Author(s) | Vishakha Agrawal |
---|---|
Country | United States |
Abstract | As deep learning models continue to grow in size and complexity, the need for efficient deployment on resource- constrained devices becomes increasingly important. We examine various compression methods including pruning, quantization, knowledge distillation, and low-rank factorization. Our analysis covers the theoretical foundations, implementation challenges, and practical trade-offs of each approach. This paper presents a comprehensive overview of model compression techniques for deep neural networks (DNNs). We also discuss emerging trends and future research directions in model compression. |
Keywords | Pruning, Quantization, Knowledge Distillation, and Low-rank factorization |
Field | Engineering |
Published In | Volume 1, Issue 3, November 2020 |
Published On | 2020-11-05 |
Cite This | An Overview of Model Compression Techniques for Deep Neural Networks - Vishakha Agrawal - IJLRP Volume 1, Issue 3, November 2020. DOI 10.5281/zenodo.14673062 |
DOI | https://doi.org/10.5281/zenodo.14673062 |
Short DOI | https://doi.org/g8z64d |
Share this

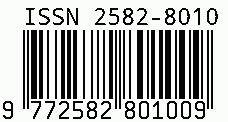
CrossRef DOI is assigned to each research paper published in our journal.
IJLRP DOI prefix is
10.70528/IJLRP
Downloads
All research papers published on this website are licensed under Creative Commons Attribution-ShareAlike 4.0 International License, and all rights belong to their respective authors/researchers.
