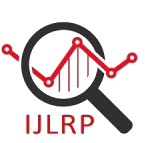
International Journal of Leading Research Publication
E-ISSN: 2582-8010
•
Impact Factor: 9.56
A Widely Indexed Open Access Peer Reviewed Multidisciplinary Bi-monthly Scholarly International Journal
Plagiarism is checked by the leading plagiarism checker
Call for Paper
Volume 6 Issue 4
April 2025
Indexing Partners

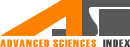
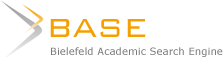

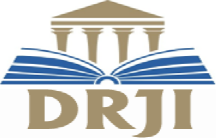

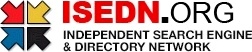

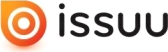

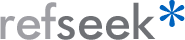
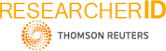
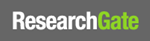
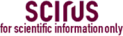
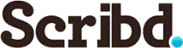

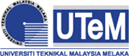
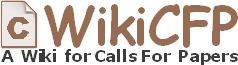
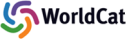
Key Challenges and Strategies in Managing Databases for Data Science and Machine Learning
Author(s) | Sethu Sesha Synam Neeli |
---|---|
Country | United States |
Abstract | The convergence of data science and machine learning (ML) methodologies with enterprise-level data management systems necessitates a paradigm shift in database administration (DBA) practices. This integration presents significant hurdles, including the need for high-throughput data storage solutions (e.g., distributed NoSQL databases, columnar databases), real-time data streaming architectures (e.g., Apache Kafka, Apache Flink), robust data governance frameworks to ensure data quality and compliance (e.g., implementing data lineage tracking, metadata management), efficient management of heterogeneous data sources via ETL/ELT processes, and optimization strategies to mitigate the performance impact of ML model deployment and inference (e.g., model caching, query optimization techniques). Addressing these challenges requires a multi-faceted approach. This includes leveraging scalable database architectures (e.g., sharding, replication), implementing automated data manipulation and transformation processes (e.g., scripting with Python, leveraging cloud-based ETL services), and enforcing stringent security protocols using encryption, access control lists (ACLs), and intrusion detection systems. Furthermore, continuous professional development is crucial, encompassing expertise in areas such as AI-driven database auto-tuning, cloud-native database services (e.g., AWS RDS, Azure SQL Database, Google Cloud SQL), and containerization technologies (e.g., Docker, Kubernetes) for deploying and scaling ML workflows. By adopting these best practices, DBAs can ensure the efficiency, reliability, and scalability of data infrastructures essential for successful data science and ML initiatives |
Keywords | DBMS, ML/AI, Scalability, Data Security & Compliance, Automation & Orchestration |
Field | Engineering |
Published In | Volume 2, Issue 3, March 2021 |
Published On | 2021-03-10 |
Cite This | Key Challenges and Strategies in Managing Databases for Data Science and Machine Learning - Sethu Sesha Synam Neeli - IJLRP Volume 2, Issue 3, March 2021. DOI 10.5281/zenodo.14672937 |
DOI | https://doi.org/10.5281/zenodo.14672937 |
Short DOI | https://doi.org/g8z63s |
Share this

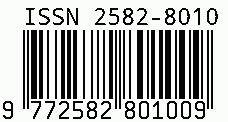
CrossRef DOI is assigned to each research paper published in our journal.
IJLRP DOI prefix is
10.70528/IJLRP
Downloads
All research papers published on this website are licensed under Creative Commons Attribution-ShareAlike 4.0 International License, and all rights belong to their respective authors/researchers.
